Neuro-Symbolic AI: Revolutionizing Precision Marketing through Hybrid Intelligence
We want to use Neuro-Symbolic AI to understand patterns and make better, faster, and cheaper decisions for marketing operations.
Michael had always been a creative marketer, but lately, it felt like he was on a sinking ship. Despite weeks of brainstorming, strategizing, and executing his latest campaign delivered a disappointingly low return on investment.
His audience, once so engaged, had grown distant, and every dollar spent on ads seemed to vanish with little impact. The numbers on his reports were a painful reminder that something had to change, and fast.
Frustrated, Michael knew he needed more than just good content or better targeting; he needed a breakthrough. That’s when he found Matrix Marketing Group’s Neuro-Symbolic AI for Precision Marketing.
Intrigued by its promise to blend human understanding with advanced AI for laser-focused campaigns, Michael decided to give it a shot—figuring he had nothing to lose.
The results were nothing short of astonishing.
Matrix’s Neuro-Symbolic AI quickly analyzed Michael’s audience, going beyond surface demographics to uncover deep insights about their behaviors, emotions, and preferences.
The technology helped Michael create personalized messages that resonated with his audience in ways he had never imagined. Each campaign became more than just an ad; it was a conversation that spoke directly to what his customers wanted and needed.
Within weeks, his metrics transformed. Engagement rates soared, cost per acquisition dropped, and his ROI began climbing back to levels he hadn’t seen in years. A renewed excitement for marketing replaced the sense of frustration and anxiety that had loomed over Michael.
With Matrix Marketing Group’s precision solution, he wasn’t just running campaigns—he was reaching people deeper, and the results spoke for themselves. Michael went from struggling to succeed to setting a new standard for marketing excellence in his company.
Introduction Neuro-Symbolic AI
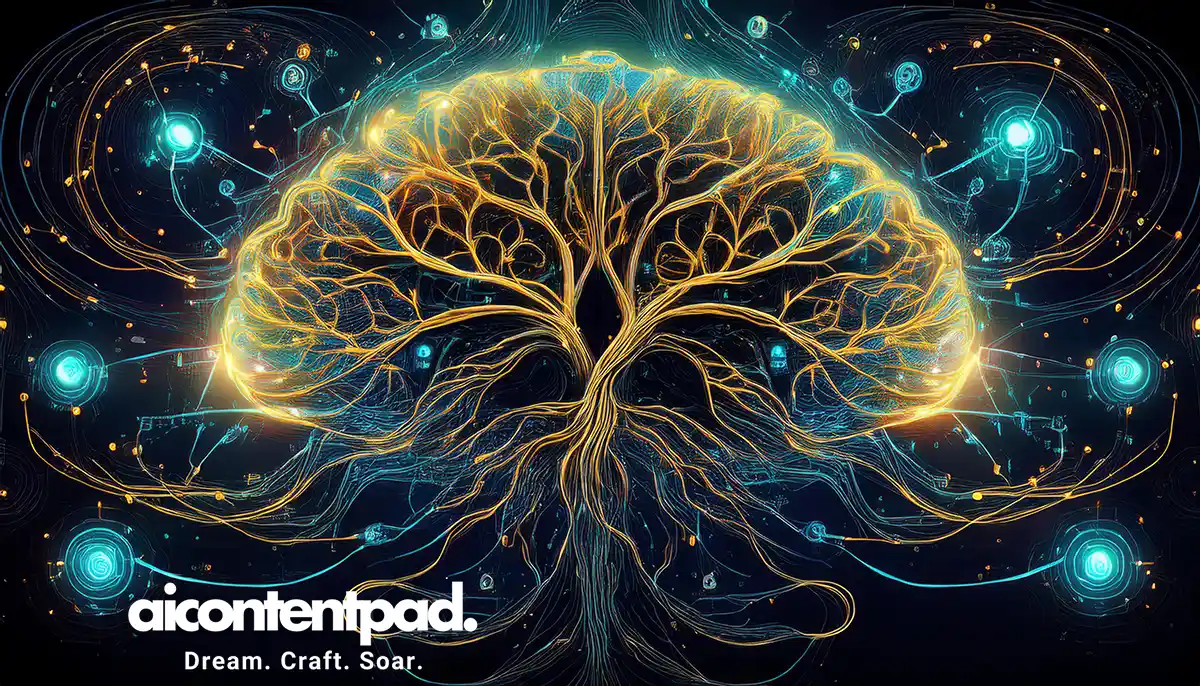
In an increasingly crowded digital marketplace, precision marketing has emerged as a key strategy for businesses aiming to target customers with the right message at the right time.
Precision marketing involves using data-driven insights to create highly personalized campaigns that resonate with individual consumers.
Focusing marketing efforts on specific audience segments enhances efficiency and effectiveness, helping businesses reduce wasted ad spend and improve overall campaign performance.
Enter Neuro-Symbolic AI, a hybrid approach that combines the strengths of neural networks and symbolic AI.
While neural networks excel at learning patterns from massive amounts of data, symbolic AI focuses on logical reasoning and explicit knowledge representation. Together, these approaches can provide a more nuanced understanding of customer behavior and preferences, driving more effective marketing strategies.
This article will explore how Neuro-Symbolic AI can significantly enhance precision marketing by enabling more accurate customer segmentation, highly personalized messaging, and optimized campaign strategies.
Understanding the Components
Neural Networks
Neural networks are a subset of machine learning inspired by the human brain. They consist of layers of interconnected nodes, or neurons, that process information like human neurons communicate.
Neural networks’ main strength is their ability to recognize complex patterns within large datasets, making them ideal for predictive tasks.
In marketing, neural networks predict customer behavior, recommend products, and identify customer segments based on past activities.
One of the most common applications of neural networks in marketing is customer profiling.
By analyzing data from previous purchases, online behaviors, and even social media interactions, neural networks can create detailed customer profiles that help marketers better understand who their customers are and what they are likely to want.
Product recommendation engines, such as those used by Amazon and Netflix, are another prime example of neural networks in action.
By learning from customer behavior, these systems can predict what products or content will interest an individual consumer, driving higher engagement and sales.
Symbolic AI
Symbolic AI, often called good old-fashioned AI (GOFAI), represents knowledge through symbols and rules. This is general AI stuff.
Unlike neural networks, which require vast amounts of data to learn, symbolic AI relies on human-defined rules and logical relationships.
This makes symbolic AI particularly useful for tasks requiring reasoning, transparency, and interpretability—critical when dealing with complex decision-making processes.
In marketing, symbolic AI can be used to create rule-based targeting strategies. For instance, marketers can use explicit rules to target customers based on predefined conditions, such as purchasing behavior or demographics.
This makes symbolic AI particularly valuable for analyzing campaigns and understanding why specific decisions were made.
By providing clear, interpretable insights, symbolic AI allows marketers to refine their strategies with a precision that is difficult to achieve with neural networks alone.
The Synergy of Neuro-Symbolic AI
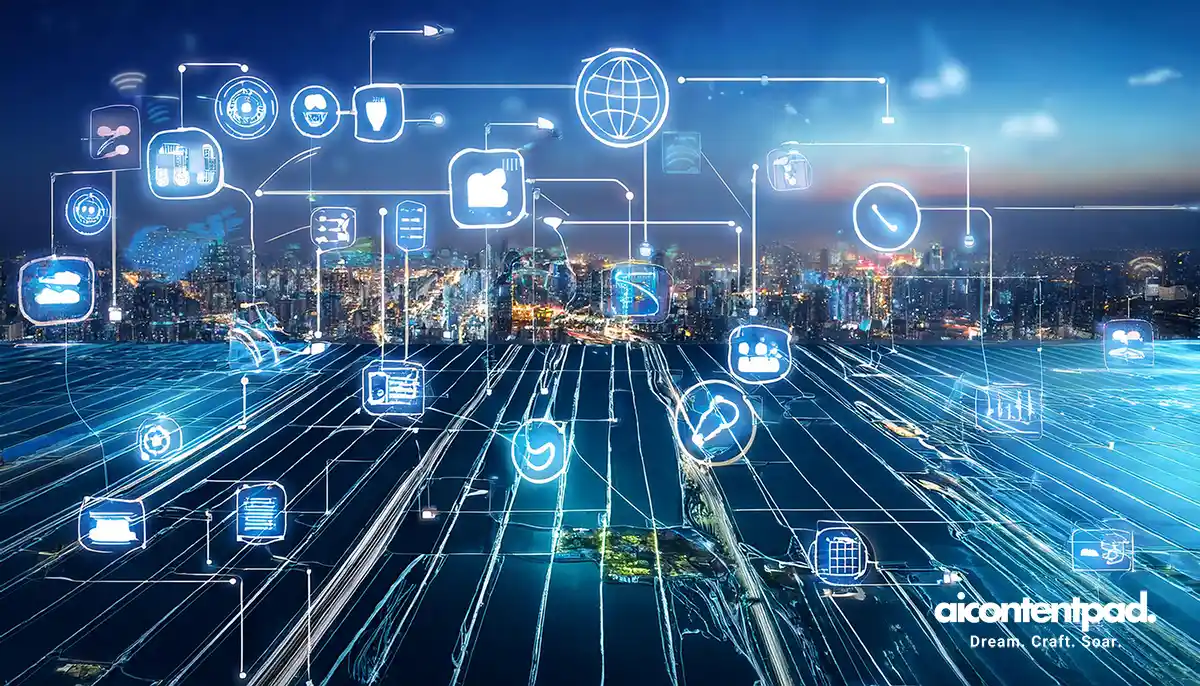
The combination of neural networks and symbolic AI creates a powerful synergy that addresses the limitations of each approach.
Neural networks are adept at handling large datasets and finding patterns that are not easily discernible by humans.
However, they often lack transparency and can be seen as “black boxes.” On the other hand, Symbolic AI provides logical reasoning and interpretability but needs help with scalability and pattern recognition in unstructured data.
Neuro-symbolic AI combines these strengths, allowing for more comprehensive decision-making processes in marketing.
Improved Customer Segmentation
One key advantage of Neuro-Symbolic AI in precision marketing is improved customer segmentation. Neural networks can identify patterns in customer behavior, such as purchasing habits or engagement preferences.
At the same time, symbolic AI can apply explicit knowledge about these behaviors to create meaningful customer segments. Combining these two approaches, neuro-symbolic AI enables more precise segmentation, ensuring marketing messages reach the most relevant audiences.
For example, a marketer might use a neural network to identify clusters of customers with similar buying patterns and then apply symbolic rules to define segments based on explicit factors like age, income, or geographic location.
Enhanced Personalization
Another critical benefit of neuro-symbolic AI is enhanced personalization. In today’s competitive market, customers expect tailored experiences that cater to their needs and preferences.
Neuro-symbolic AI can analyze implicit behavioral data, such as browsing history, and explicit information, like customer feedback or stated preferences.
By integrating these insights, marketers can craft relevant, personalized messages that resonate with consumers on a deeper level.
For instance, Neuro-Symbolic AI can determine which product recommendations are most appropriate based on behavioral patterns and logical customer data, such as purchase frequency or stated interests.
Optimized Campaign Performance
Optimizing campaign performance is another area where Neuro-Symbolic AI can significantly impact.
By dynamically adjusting campaigns based on real-time data, Neuro-Symbolic AI ensures that marketing initiatives remain effective and aligned with predefined business objectives. Neural networks can monitor key real-time performance indicators (KPIs), identifying trends and anomalies.
At the same time, symbolic AI can apply logical rules to make sense of this data and suggest actionable adjustments.
This combination enables marketers to fine-tune their campaigns on the fly, maximizing return on investment (ROI) and ensuring that marketing messages continue to resonate with their intended audiences.
MatrixLabX is pushing the boundaries of artificial intelligence by implementing neuro-symbolic AI in its Branch Models, blending the strengths of symbolic reasoning with the power of neural networks. This approach allows MatrixLabX to tackle some of the most complex marketing challenges by integrating the precision of human-like reasoning with the flexibility and scalability of deep learning.
What is Neuro-Symbolic AI?
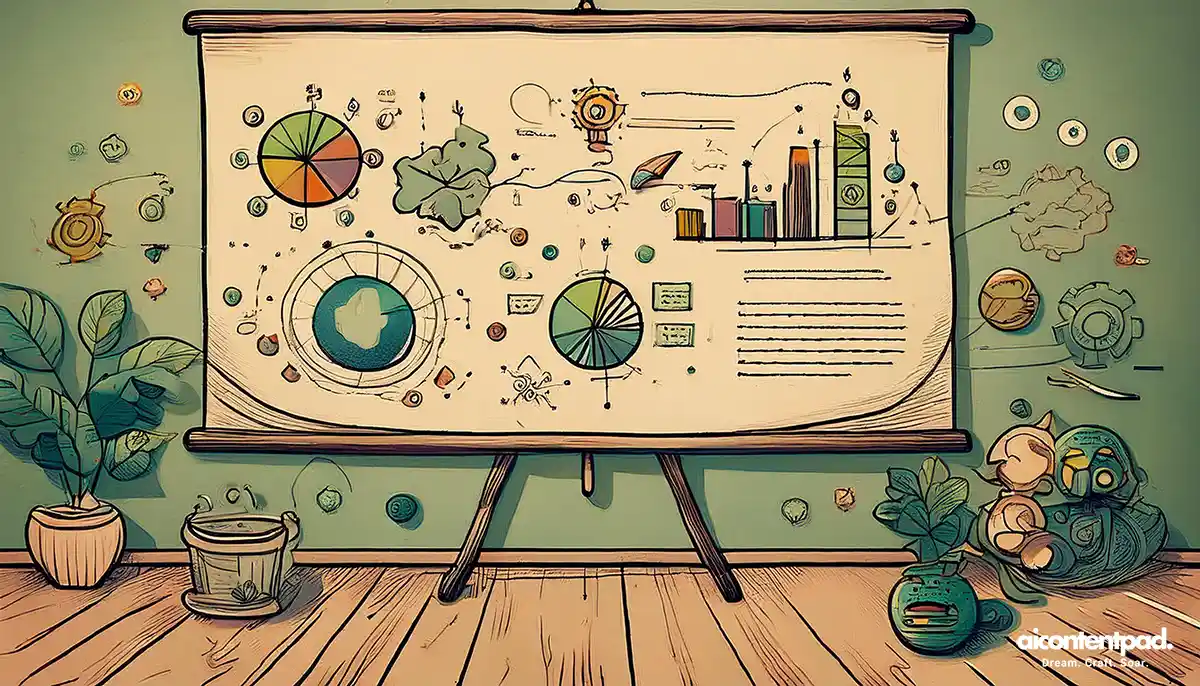
Neuro-symbolic AI is an approach that combines neural networks (which excel in pattern recognition, learning from vast data sets, and extracting subtle features) with symbolic AI (which excels in logic, rules, and structured problem-solving).
This hybrid approach enhances AI’s reasoning ability while remaining flexible enough to deal with real-world complexity, making it ideal for nuanced marketing scenarios.
Here’s an analogy that might help marketing managers grasp the concept of neuro-symbolic AI:
Think of neuro-symbolic AI as the perfect combination of a seasoned marketing strategist and industry marketing expert working together.
- The seasoned marketing strategist (neural networks) is excellent at spotting trends and making sense of large volumes of social media data, customer feedback, and performance metrics. They might not fully understand the deeper reasons behind those trends, but they’re fantastic at picking up on patterns and connections that might be hard to see otherwise.
- On the other hand, the seasoned industry marketing expert (symbolic AI) has years of experience and knows all the rules, strategies, and insights that come with deep market knowledge. They can explain why a specific approach works, add context, and apply logical reasoning to create meaningful campaigns.
When the marketing strategist and the industry marketing expert work together, the professional marketing strategist can quickly point out emerging trends, and the professional industry marketing expert can decide the best way to use that information.
Today, a marketing operation is at a steady state. It may see incremental improvements over time, but with Matrix’s neuro-symbolic AI embedded in the AI agents and Branch Models, MatrixLabX is at the top of marketing technology innovation.
This is how neuro-symbolic AI works: combining the data-driven insights of the neural network with the logical expertise of symbolic AI.
Together, they can tell you what’s happening and why it’s happening—and how you can turn that knowledge into a winning marketing strategy.
This blend leads to more targeted campaigns, smarter customer insights, and a better market understanding. It allows you to make data-backed decisions with clear reasoning behind each move.
Application in MatrixLabX Branch Models
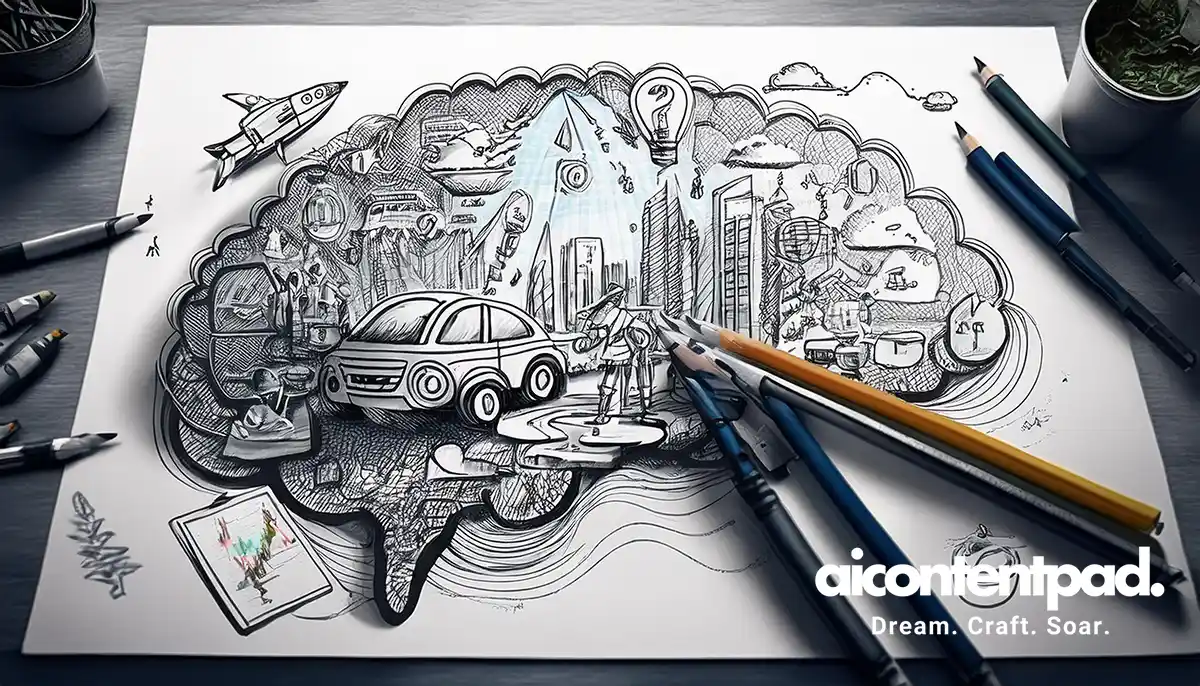
- Hybrid Decision Making: MatrixLabX uses neuro-symbolic AI to enhance its Branch Models’ decision-making capabilities. The neural networks help the models learn from enormous datasets—customer behavior data, market trends, and competitor analysis—while the symbolic logic layer applies rules and constraints. This combination allows Branch Models to understand the why behind data patterns, making recommendations that are not only data-driven but also logically sound.
For instance, if the neural network identifies a pattern of increased engagement among a particular demographic in response to a certain ad type, the symbolic AI layer can reason that the engagement may be due to cultural factors. It can even propose rule-based recommendations, such as adapting the campaign content to fit that cultural context. - Content Personalization: MatrixLabX uses neuro-symbolic AI for hyper-personalized content creation. The neural aspect detects the preferences and behavior of individual users from data patterns, and the symbolic AI applies logical structures to create contextually relevant content. With our systems in place, an e-commerce brand wants to launch a targeted marketing campaign. The neural network analyzes previous purchasing data and user behaviors, while the symbolic layer reasons user intent—determining whether someone is buying out of necessity, a gift for a holiday, or another occasion. This deep understanding makes content generated by the Branch Models much more relatable and targeted, driving higher engagement rates.
- Complex Query Handling: MatrixLabX Branch Models also leverage neuro-symbolic AI for complex query handling, especially in customer interactions. Neural networks first parse and understand the customer query’s language nuances, and the symbolic AI then performs logical operations to extract meaning and deliver precise, reasoned responses. For instance, when a marketing manager asks how a particular campaign performed compared to similar campaigns, the neuro-symbolic AI can analyze this complex query by applying data-driven insights and logical reasoning. It can explain why a particular campaign performed better, like attributing success to unique creative elements or timing of ad delivery.
- Branch Learning with Symbolic Knowledge Injection: Neuro-symbolic AI enables MatrixLabX’s Branch Models to integrate domain-specific knowledge. Symbolic AI allows the embedding of existing marketing frameworks and business logic directly into the Branch Models, accelerating their learning curves. For instance, when expanding into new markets, the Branch Models can integrate rules and guidelines about cultural nuances, legal regulations, and marketing strategies that have been encoded symbolically. The neural network then adjusts these abstract rules dynamically based on incoming data about that market’s response. This hybrid learning model allows MatrixLabX to adapt swiftly to new environments while respecting important structural rules of the industry.
- Enhanced Interpretability: One criticism of traditional neural networks is their “black box” nature, which makes it hard to interpret how decisions are made. Neuro-symbolic AI helps overcome this problem, as the symbolic reasoning layer allows MatrixLabX Branch Models to explain their decision-making processes more transparently.
This means marketers can understand the rationale behind an AI-generated marketing strategy—such as why certain audiences were chosen for a campaign or specific creative elements are expected to perform better.
This level of interpretability builds trust with marketing managers and helps fine-tune strategies for optimal performance.
Real-World Example: Campaign Strategy Optimization
MatrixLabX recently utilized neuro-symbolic AI to optimize a campaign across several branches of a global retail brand. Here’s how it worked:
- Pattern Recognition: The neural networks analyzed sales and engagement data across multiple geographies, identifying trends and behaviors that were not immediately visible through traditional analysis.
- Reasoned Strategy Adaptation: Symbolic AI then combines reasoning rules based on market-specific guidelines, such as cultural holidays, consumer sentiment trends, and product relevance.
- Implementation and Iteration: The hybrid approach allowed the Branch Models to create personalized marketing strategies for each geographic branch, iteratively improving results as more data was gathered during the campaign’s progress.
Benefits for MatrixLabX
- Scalable Yet Tailored Solutions: Neuro-symbolic AI provides scalability similar to neural networks while allowing for tailored, context-aware strategies. MatrixLabX can deploy domain-specific AI agents running Branch Models AI solutions across different branches and industries, each benefiting from customized logic and local market nuances.
- Efficient Data Utilization: Neuro-symbolic AI models can better leverage sparse data or generalize from smaller datasets than pure neural networks. The symbolic component helps reason and bridge gaps when data is limited, which is crucial for emerging markets or industries with limited historical data.
- AI-Guided Marketing Insights: MatrixLabX Branch Models provide outputs and explain why certain actions are recommended. This helps clients make informed decisions and better understand the underlying strategy.
MatrixLabX continues to advance its neuro-symbolic AI capabilities to enhance its Branch Models further. The future focus includes:
- Deepening Symbolic Integration: By incorporating more domain-specific rules and expanding the symbolic logic base, MatrixLabX aims to make its models even more insightful, particularly for highly regulated industries.
- Adaptive Logic Learning: Introducing mechanisms for Branch Models to learn new symbolic rules dynamically based on data, helping MatrixLabX evolve and adapt logical reasoning alongside its neural learning.
MatrixLabX’s use of neuro-symbolic AI for its Branch Models combines the best of both worlds: data-driven pattern recognition and human-like logical reasoning.
This hybrid approach allows for more sophisticated decision-making and content personalization and provides a transparent, reasoned explanation behind each strategy.
As a result, MatrixLabX is well-positioned to deliver more insightful, adaptive, and effective AI-driven marketing solutions that cater to the diverse needs of global and local markets alike.
Real-world Applications and Case Studies
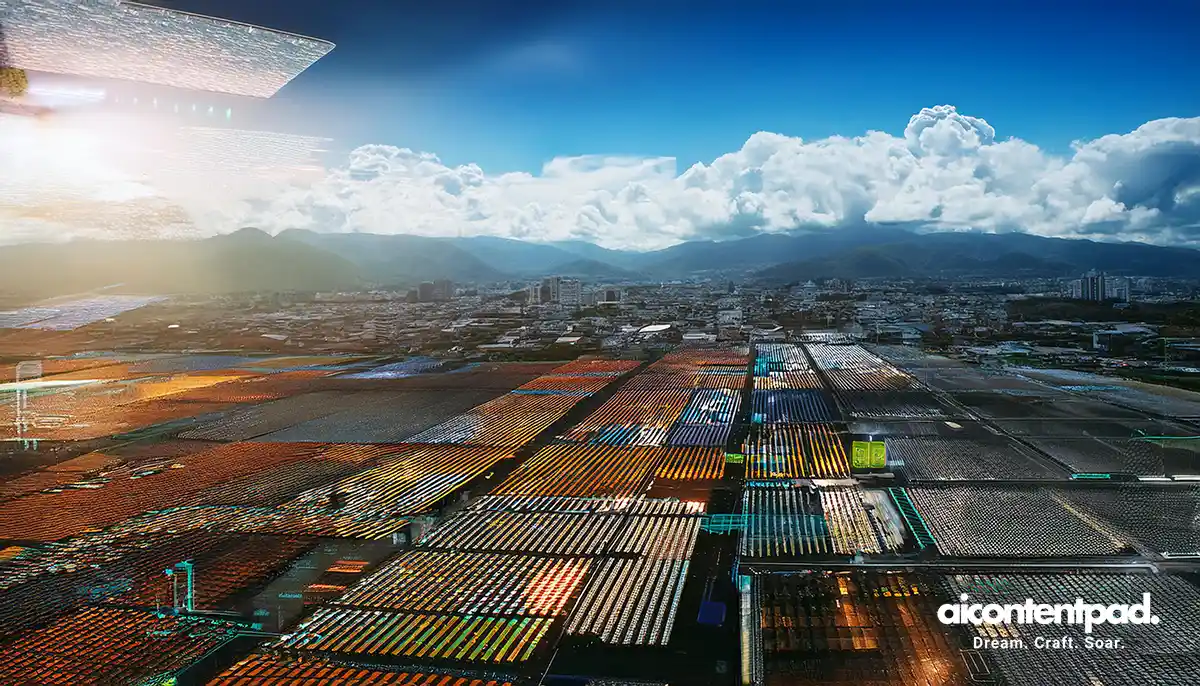
Several companies have already begun to leverage Neuro-Symbolic AI to enhance their precision marketing efforts. One notable example is IBM, which has been a pioneer in the field of Neuro-Symbolic AI through its Watson platform. We witnessed this in 2011 with the Jeopardy Challenge.
IBM Watson combines neural networks’ pattern recognition capabilities with symbolic AI’s logical reasoning to provide businesses with actionable insights.
In precision marketing, IBM Watson has helped brands analyze customer data more effectively, improving segmentation and personalization.
Another example is Unilever, which has used Neuro-Symbolic AI to optimize its digital advertising campaigns.
By integrating neural networks for behavioral analysis with symbolic AI for campaign logic, Unilever increased its ad targeting accuracy, resulting in higher engagement rates and better conversion outcomes.
The combination of deep learning and rule-based reasoning allowed Unilever to identify key customer segments and tailor its messaging to meet the specific needs of each group.
A smaller yet equally impactful example comes from a startup specializing in e-commerce. This company used Neuro-Symbolic AI to enhance its product recommendation system.
By analyzing both behavioral data and explicit product attributes, the company was able to offer more relevant recommendations to its customers.
As a result, the startup saw a significant increase in average order value and customer satisfaction, demonstrating the potential of Neuro-Symbolic AI to drive business growth in a highly competitive market.
Challenges and Future Directions
While Neuro-Symbolic AI holds significant promise for precision marketing, some challenges must be addressed for widespread adoption. One of the main challenges is data integration.
Neuro-symbolic AI requires large volumes of structured and unstructured data to function effectively, and integrating these disparate data sources can be a complex and time-consuming process.
Marketers must ensure that their data is clean, consistent, and accessible to fully leverage the capabilities of Neuro-Symbolic AI.
Another challenge is model interpretability. While symbolic AI is inherently interpretable due to its rule-based nature, neural networks are often viewed as black boxes.
Combining these two approaches can make it even more difficult to understand how decisions are made, which can be a barrier for marketers who need to justify their strategies to stakeholders.
Developing tools and techniques that improve the transparency of Neuro-Symbolic AI models will be essential for fostering trust and enabling broader adoption.
Despite these challenges, the future of Neuro-Symbolic AI in precision marketing looks bright. Researchers are actively improving data integration techniques, making combining structured and unstructured data sources easier.
Advances in explainable AI (XAI) are also helping to address the interpretability challenge by providing insights into how neural networks arrive at their conclusions.
As these technologies evolve, the adoption of Neuro-Symbolic AI in marketing will likely grow, providing businesses with even more powerful tools for reaching their target audiences.
AIContentPad for Enterprises
AIContentPad helps reduce high customer acquisition costs (CAC) by automating content creation for SEO-optimized blogs, social media, ads, and videos. It enables marketing managers to produce high-quality, targeted content faster and at a lower cost. This efficient content strategy attracts more organic traffic and improves conversion rates, minimizing reliance on expensive paid advertising.
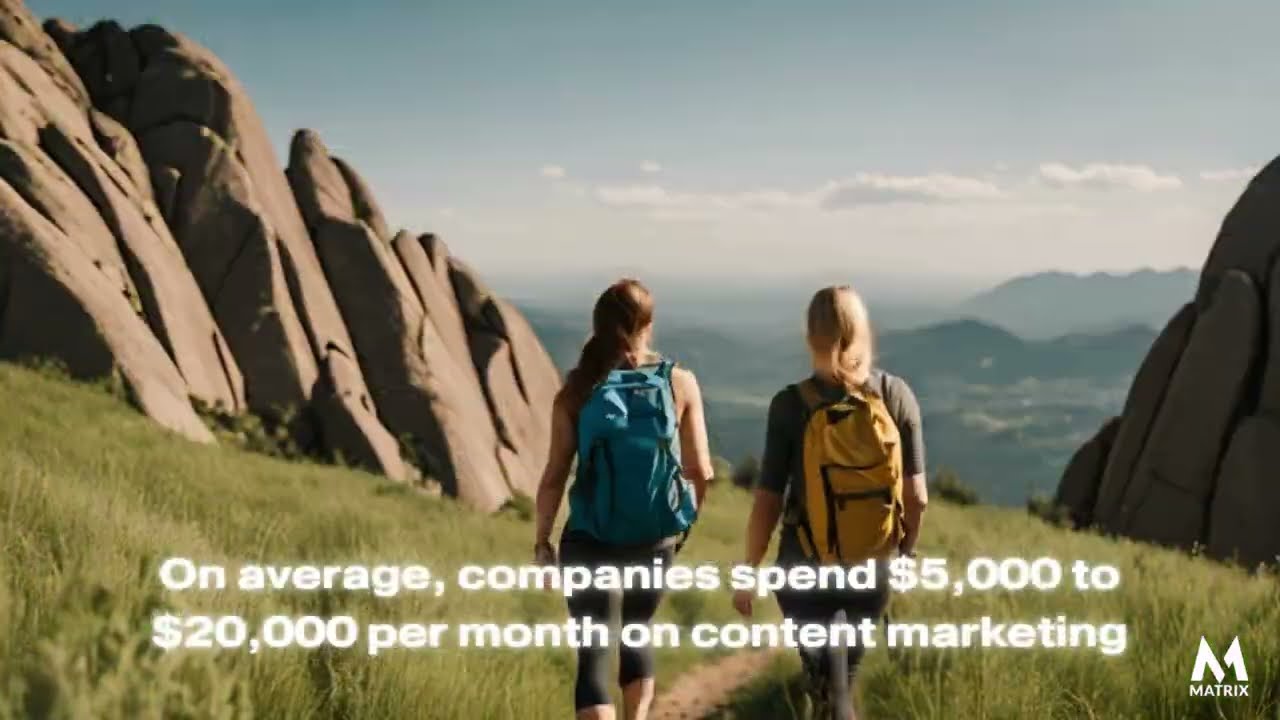
Conclusion
Neuro-symbolic AI represents a transformative approach to precision marketing, offering a unique combination of deep learning and logical reasoning.
By leveraging the strengths of neural networks and symbolic AI, marketers can create more accurate customer segments, deliver highly personalized messages, and optimize their campaigns in real-time.
While challenges such as data integration and model interpretability remain, the potential benefits of neuro-symbolic AI are undeniable.
As businesses continue to seek more effective ways to connect with their target audiences, Neuro-Symbolic AI is poised to play a pivotal role in shaping the future of marketing.
Combining the best of both worlds—pattern recognition and logical reasoning—this hybrid approach offers a powerful solution for enhancing precision marketing and delivering meaningful customer experiences. The future of AI in marketing is bright, and Neuro-Symbolic AI is at the forefront of this exciting transformation.
Further reading:
- Meta-Cognition in AI: The Next Frontier for Business Intelligence
- Balancing Speed and Precision: Lessons from IBM’s SOFAI for AI-Powered Business Decisions
- How Neuro-Symbolic AI Solves Hallucinations and Why It Matters for Your Business
- The Rise of Hybrid AI: Leveraging GNNs for Business-Ready Explainable AI
- Towards AGI: How Neuro-Symbolic AI is Bridging the Gap to Human-Like Intelligence
- Overcoming the Computational Hurdle in Neuro-Symbolic AI: Is It Worth the Investment?
- Graph Neural Networks and Symbolic AI: Shaping the Future of Knowledge-Driven Organizations
- AI Planning for Real-World Complexity: Insights from Plan-SOFAI