Undoubtedly, artificial intelligence (AI) – and its offshoot, machine learning (ML) – has emerged as a rising star on the global technology stage.
AI-powered initiatives are immense, leaving us both in awe and a little uneasy. As if a tsunami, AI and ML are radically reshaping how we engage with and navigate the world.
/im
70% of agencies need help integrating AI solutions.
70% of agencies need help integrating AI solutions into their tech stack, citing a lack of interoperability as a major barrier. 85% of marketing agencies need more in-house expertise to manage and deploy multi-AI agent systems effectively.
What is product development?
Product development is the process of building a new product from ideation to launch. It begins with those initial brainstorming sessions when you discuss a budding idea.
From there, the process is creative but strategic, and you may have seen it done in a million different ways. However, it can be easier to mesh creativity and strategy effectively with clear organization.
This is where the product development process comes in—a seven-step framework that helps you standardize and define your work.
Given the broad range of industries and the vast number of products launched yearly, listing all the product failures over the past 10 years would be difficult. However, I can provide some notable product failures from various sectors from 2013 to 2023. AI Product Management changed the process by bringing products to market quickly and better.
OrchestraAI Marketing Platform – WATCH
OrchestraAI utilizes a compound AI agent architecture as an AI Agentic Platform. This architecture seamlessly integrates multiple specialized AI agents into a cohesive system, enabling it to tackle complex, multifaceted marketing tasks.
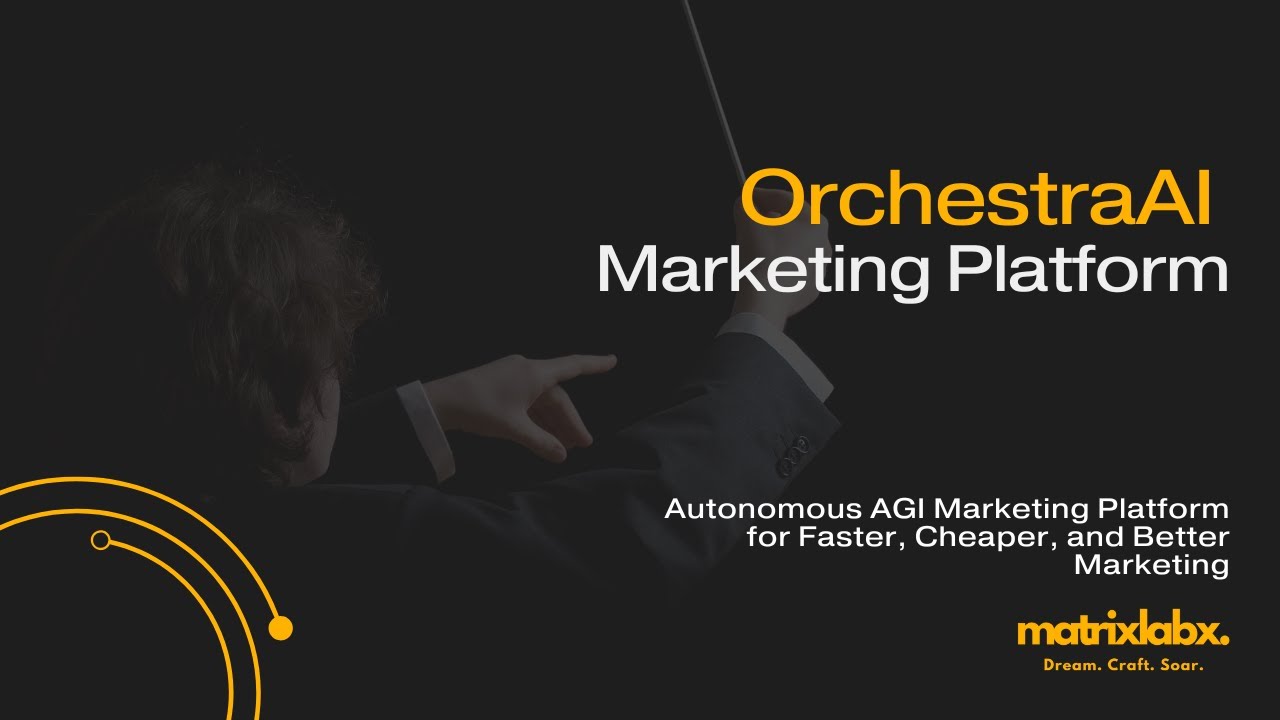
Please note that not all of these are complete failures. Some may have been commercially unsuccessful but positively impacted the company’s learning and future product development.
- Google Glass (2013): This wearable tech device was meant to be the next big thing but failed because of its high cost, privacy concerns, and lack of clear usefulness for average consumers.
- Amazon Fire Phone (2014): Despite Amazon’s success in many areas, their smartphone was a failure, primarily due to the high competition in the market, lack of standout features, and a closed ecosystem that only attracted a few users.
- Microsoft Band (2014-2016): Microsoft’s attempt at a fitness tracker needed to catch on. After two iterations, the company discontinued the product due to a lack of market interest and stiff competition.
- People (2015): Known as the “Yelp for people,” this app faced immediate backlash for its concept of allowing people to rate others as they would businesses, raising severe privacy and ethical concerns.
- Juicero (2016-2017): The Juicero press was a Wi-Fi-connected juicer that used proprietary, pre-packaged fruits and vegetables. It was criticized for its high price and the fact that the packets could be squeezed by hand, rendering the device unnecessary.
- Samsung Galaxy Note 7 (2016): This phone model became infamous for battery issues that caused the device to overheat and catch fire, leading to a full recall and discontinuation.
- GoPro Karma Drone (2016-2018): GoPro’s venture into drones was plagued by a recall due to power failures. Eventually, GoPro decided to exit the drone market altogether.
- Essential Phone (2017): Despite its high-profile creation by Android co-founder Andy Rubin, it failed to gain traction in the competitive smartphone market due to its high price and lack of standout features.
- MoviePass (2011-2019): While not strictly a product, this service deserves mention. Offering virtually unlimited movie tickets for a monthly fee, the business model proved unsustainable, and the company folded in 2019.
- Facebook’s Libra/Diem (2019-): This cryptocurrency project faced regulatory pushback from governments worldwide due to concerns about potential misuse in money laundering and the destabilization of traditional currencies.
- AGI – in 2024 with compound marketing agent systems.
These are just a few examples. The reasons for product failures are varied, from lack of market need, misjudgment of the market situation, poor marketing strategy, and technical flaws to regulatory issues. AI Agentic Systems for Marketing Healthcare Organizations
Each case provides valuable lessons for businesses on understanding consumer needs, conducting thorough market research, and regularly testing and improving their products.
Create a product development template.
Is product development the same as product management or AI product management?
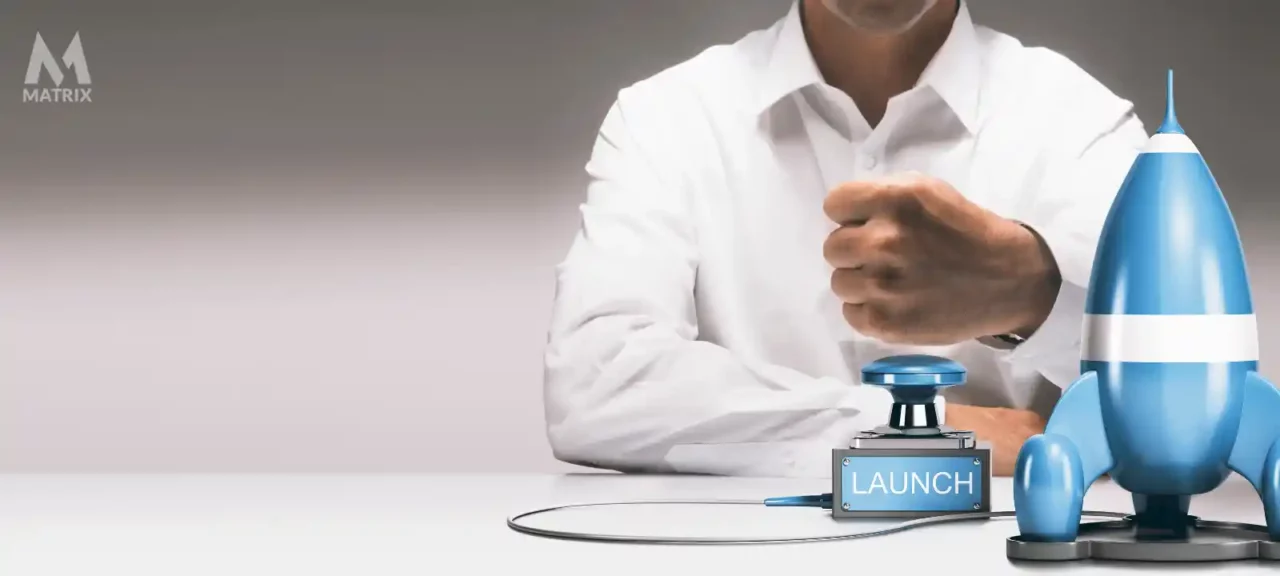
Though they sound almost identical, there’s an important difference between product development and product management.
Product development describes building a product, where product management oversees that work. It’s a slight difference but an important distinction.
A product manager who often oversees a team in the product development process will lead product management. The new AI product manager must know:
- AI product manager
- AI product strategy
- AI product development
The Skill Gap: Why 85% of Marketers Are Not Ready
The Skill Gap: Why 85% of Marketers Are Not Ready highlights the urgent need for upskilling in a rapidly evolving digital landscape. Advanced technologies like AI, data analytics, and automation are outpacing traditional marketing expertise, leaving most professionals unprepared to adapt and compete effectively.
A utopia for a product manager:
- Understanding Users: They would have real-time and in-depth insight into user needs, wants, and behaviors. User feedback would be direct, honest, and constructive, allowing for effective iterations of the product.
- Streamlined Processes: Processes from ideation to launch would be efficient and seamless. Bureaucracy would be minimal, and getting approval from stakeholders would be smooth and fast.
- Perfect Collaboration: There would be excellent collaboration between all the teams involved—engineering, design, marketing, sales, customer service, etc. A shared understanding of the product vision would lead to alignment in goals and actions. Communication would be clear, with no misunderstandings or misinterpretations.
- Ample Resources: There would be sufficient resources for all aspects of product development, from research and development to marketing and customer support.
- Cutting-Edge Technology: They would have access to the latest technology to develop, test, and launch products.
- Empowered Team: The team members would be highly skilled, motivated, and empowered to make decisions. They would have a shared passion for the product and a deep commitment to its success.
- Market Success: The product would have a clear product-market fit. The launch would be successful, with positive user reviews and high adoption rates.
- Clear Vision: The product strategy and roadmap would be clear, well-articulated, and easily understood by everyone involved. There would be a balance of short-term wins and long-term vision.
- Work-Life Balance: The product manager would be able to maintain a healthy work-life balance with no excessive stress or burnout.
- Continuous Learning and Improvement: A culture of continuous learning and improvement would exist where mistakes are seen as opportunities for learning rather than failures.
While this may be a product manager’s utopia, it’s important to remember that challenges and obstacles are inevitable in any job. What’s more important is being adaptable, resilient, and continually striving for improvement. AI and product management go hand in hand, from market research to writing product decisions and web copy. AI Product Management can help!
We use an AI-driven product development process to get products to market faster. AI Blog Post Generation GPT Agent: Unleash the Future of Content Creation
An AI-driven Product Manager (PM) in a startup technology company would have a role similar to a traditional PM but with added responsibilities and knowledge requirements specific to artificial intelligence products. Here are some potential responsibilities:
- Product Vision & Strategy: As in any PM role, an AI PM needs to set a strategic direction for the product that aligns with the startup’s goals and market opportunities. However, they must also understand AI’s unique possibilities and limitations and incorporate these into their product vision and strategy.
- Understanding of AI: The AI PM needs to have a good understanding of AI technologies, including machine learning algorithms, data science, neural networks, and more. They don’t necessarily need to be able to build AI systems themselves, but they should understand how they work, how they can be used, and their strengths and weaknesses.
- Data Management: Since AI technologies rely heavily on data, an AI PM needs to understand data management well. They must work closely with data scientists and engineers to collect and use the right data correctly.
- Cross-Functional Collaboration: As with any PM, an AI PM needs to coordinate with various functions within the startup. However, they must also facilitate communication between AI specialists (like data scientists) and non-specialists to ensure everyone understands the product and works towards the same goals.
- Ethics and Compliance: AI products can raise unique ethical and compliance issues, from data privacy concerns to the risk of algorithmic bias. The AI PM must be aware of these issues and incorporate them into product development.
- User Experience: AI can sometimes behave in ways that are hard for users to understand or predict. The AI PM needs to create a user experience that makes the AI’s behavior transparent and understandable and builds user trust.
- Validation and Testing: Due to AI’s unpredictable nature, testing an AI product can be more complex than testing a traditional software product. The AI PM needs to ensure that the product is tested thoroughly and its performance is validated before release.
- Product Launch & Marketing: When launching and marketing an AI product, the PM needs to be able to explain the benefits of the AI in a way that is easy for potential customers to understand without overhyping its capabilities.
In an AI startup, the PM plays a crucial role in bridging the gap between complex AI technology, users’ needs, and market needs. They need to be technically savvy but also able to think from a business and user perspective.
Product development processes vary based on the nature of the product, the team, and the organization’s culture.
Here are five commonly used product development methodologies in technology companies, along with their advantages, disadvantages, and comparison with an AI-driven product development process:
1. Waterfall Model:
The waterfall model is a traditional way of product development. It involves creating a list of requirements, designing the architecture and functionality, coding the product, and testing and debugging it before deploying. This process has been slow and tedious, as each step needs to be completed sequentially.
2. Agile Model:
The agile model emphasizes an iterative approach during which changes can be made easily and quickly. It is divided into sprints, which enables teams to consistently deliver smaller chunks of their product early on and get feedback from users so they can decide whether to continue or pivot.
Design thinking is a user-centric approach that heavily relies on understanding customers’ needs through research and experimentation. This method helps teams focus on creating.
- Advantages: Clear structure, easy to understand and manage, well-defined stages and deliverables.
- Disadvantages: Not flexible, changes are hard to implement once the development has started, and there are long development cycles.
- In an AI context, The rigid structure of Waterfall can be a disadvantage given the exploratory and iterative nature of AI development. AI requires the flexibility to refine models based on feedback, which might not be compatible with Waterfall’s sequential structure.
2. Agile Methodology:
Agile Methodology is an iterative and incremental approach to software development. It encourages teams to be customer-centric, work in shorter sprints, regularly review progress, and adjust plans as needed.
Advantages: It offers faster time to market, more flexibility for changes during the development process, and better control over costs.
Disadvantages: Includes
- Advantages: It is highly flexible and adaptable, encourages frequent communication and feedback, and uses short development cycles (sprints) for quicker delivery.
- Disadvantages: Can be chaotic without proper management, has less predictability, and requires high customer involvement.
- In an AI context, Agile can well suit AI development due to its iterative nature. It allows for the flexibility to refine AI models based on new data or feedback.
3. Scrum:
Scrum is an agile methodology that divides work into smaller, manageable chunks called “sprints.”
- Advantages: This highly structured approach, with clear roles and responsibilities, allows for fast development cycles and encourages team collaboration.
- Enhances team productivity, delivers high-quality software rapidly, and provides a framework for addressing complexity.
- Disadvantages: Requires experienced and committed team members, may lead to scope creep if not managed carefully, and can be intensive for team members.
- In an AI context, Scrum’s iterative approach can benefit AI development. Regular meetings (like daily stand-ups and sprint reviews) can help monitor progress and refine models or approaches.
4. Lean Development:
Lean Development is an approach to reducing waste in the software development process. It emphasizes delivering customer value and building fewer features with faster feedback loops.
- Advantages: Minimizes waste by creating value for the customer, encourages continuous improvement, and emphasizes fast delivery.
- Disadvantages: Requires a high level of discipline and understanding, can lead to team burnout if not managed properly, and risks oversimplifying the process.
- In an AI context, Lean’s principle of eliminating waste can help create AI models by focusing only on what brings value to the end user. However, the emphasis on speed might overlook the complexities of AI development.
5. Kanban:
Kanban is a project management methodology that emphasizes transparency, flexibility, and visual clarity. It helps teams work efficiently and effectively toward their goals.
- Advantages: Visualizes workflow, promotes continuous delivery, reduces waste, and flexibility in planning.
- Disadvantages: This can lead to overemphasizing individual tasks rather than overall progress. Needs to work better with big, long-term projects.
- In an AI context, Kanban can help manage the AI development workflow, especially data pipelines or model improvement tasks. However, it may need to capture the complexity and interdependencies of AI tasks.
So, have you seen I have integrated AI into these processes? However, if you move into the software that supports these processes, artificial intelligence still needs to be integrated into the system. If we look at Microsoft, HubSpot, many others are stitching it in. but that’s only a Band-Aid.
Choosing the right product development process depends on the product’s nature and the organization’s context. Due to AI development’s exploratory and uncertain nature, an iterative and flexible approach like Agile or Scrum might be more effective for AI-driven product development.
These methodologies allow for regular feedback and continuous improvement, which is crucial for refining AI models and ensuring they deliver value to the end user. They also encourage close collaboration and communication among team members, which can help bridge the gap between AI specialists and non-specialists.
However, it’s important to note that no methodology is perfect, and the choice of methodology should be adapted to the specific needs and context of the project and the team. The effectiveness of any methodology also depends on how well it’s implemented and how well the team adheres to its principles.
Transforming Product Management with AI: The Future is Here
Introducing OrchestraAI, an AI-first product management system. This groundbreaking platform is designed to revolutionize how businesses approach product management.
Unlike traditional methods that rely on manual data collection, siloed decision-making, and labor-intensive processes, OrchestraAI leverages cutting-edge artificial intelligence to streamline, optimize, and elevate every aspect of product management. What is Neuro-Symbolic AI for Precision Marketing?
Why OrchestraAI Outperforms Traditional Product Management
- Data-Driven Precision:
OrchestraAI analyzes vast datasets in real-time, identifying market trends, customer preferences, and performance metrics faster and more accurately than human teams. Traditional methods are often limited by time and resources, leading to decisions based on incomplete or outdated information. - Enhanced Collaboration: Forget the bottlenecks of traditional systems, where communication breakdowns across teams delay progress. OrchestraAI is an AI-powered tool that ensures seamless collaboration by integrating workflows, aligning priorities, and providing a unified platform for cross-functional teams.
- Accelerated Time-to-Market: While manual approvals and outdated frameworks weigh down traditional product management processes, OrchestraAI automates repetitive tasks and prioritizes actionable insights, enabling faster iteration cycles and quicker launches.
- Proactive Risk Management: Predict potential roadblocks before they become problems. OrchestraAI’s predictive analytics enable proactive decision-making, reducing costly errors that traditional approaches often fail to anticipate.
- Personalized Customer Experiences: OrchestraAI doesn’t just manage products; it helps design them. By analyzing customer feedback and usage patterns, the system suggests innovative features tailored to specific audience needs, ensuring better product-market fit than ever before.
Key Features of OrchestraAI
- Smart Roadmap Generation: Automatically generate and update product roadmaps based on evolving data.
- AI-Driven Prioritization: Focus resources on high-impact opportunities with advanced prioritization algorithms.
- Seamless Integration: Connect with existing tools and platforms to maintain continuity without disruption.
- Continuous Optimization: OrchestraAI learns and evolves with your product, fine-tuning strategies for long-term success.
The Verdict
Traditional product management served its purpose in the pre-digital era. But as markets evolve, OrchestraAI is the key to staying competitive. By eliminating inefficiencies, empowering data-driven decisions, and fostering innovation, Symphony sets a new gold standard for product management.
Ready to embrace the future of product management? Let OrchestraAI lead the way.
The 7 Stages of Product Development
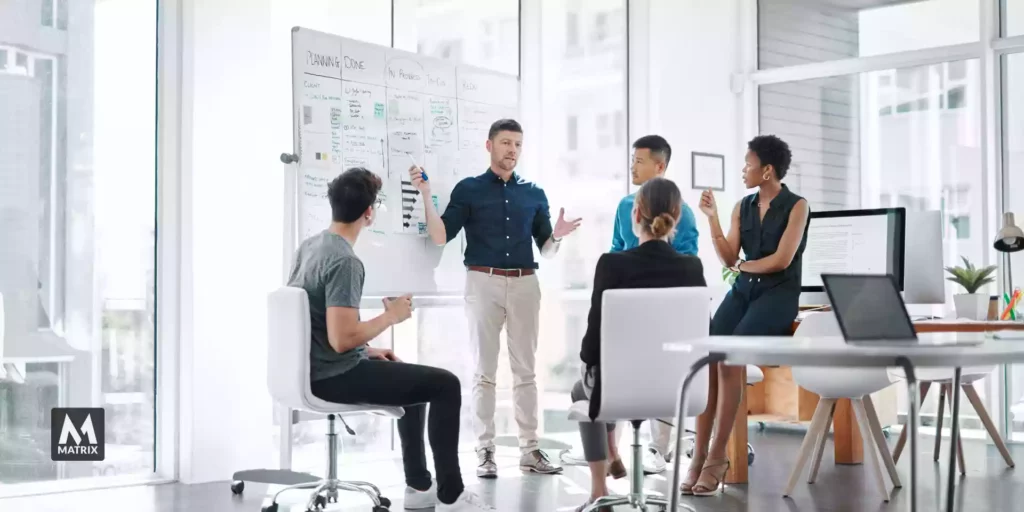
The product development process helps simplify a launch and encourages cross-team collaboration, with teamwork and communication at the forefront of the process.
Let’s explore the product life cycle and define the six product phases, all of which can help you successfully launch your next product.
The number of stages in the new product development process can vary depending on the specific product and industry. However, there are typically 5-7 main stages:
- Ideation: This stage generates new product ideas. It can involve brainstorming, market research, or customer interviews.
- Validation: Once a few promising ideas have been generated, they need to be validated to see if there is a market for them. This can be done through surveys, focus groups, or A/B testing.
- Prototyping: Once an idea has been validated, it is time to create a prototype. This working product model can be used to test the functionality and usability.
- Testing: The prototype is then tested with users to gather feedback, which can be used to improve the product before it is launched.
- Development: Once the product is finalized, it is time to start development. This involves the actual manufacturing or creation of the product.
- Launch: Once the product is developed, it is time to launch it on the market. This involves marketing and sales activities to reach potential customers.
- Post-launch: The product development process continues after launch. It is important to monitor the product’s performance and make changes as needed.
The specific stages of the new AI product development process may vary depending on the product and industry. However, the overall goal is to create a product that meets the target market’s needs and is successful in the marketplace. What is an AI Content Assessment?
Here are some additional stages that may be included in the new product development process:
- Business analysis: This stage involves assessing the product’s feasibility from a business perspective. This includes factors such as the market size, the target audience, and the competition.
- Marketing: This stage involves developing a marketing plan for the product. This includes identifying the target audience, creating marketing materials, and developing a sales strategy.
- Finance: This stage involves securing the funding needed to develop and launch the product. This may involve seeking investment from venture capitalists or angel investors.
The new product development process can be long and complex. However, following the steps outlined above can increase your chances of success.
There are many places where time can be compressed in the new product development process.
Here are a few examples:
- Ideation: This is the stage for generating new product ideas. Using tools like market research and customer interviews, you can quickly identify potential product ideas that will likely succeed. AI can help here.
- Validation: Once a few promising ideas have been generated, they need to be validated to see if there is a market for them. This can be done through surveys, focus groups, or A/B testing. Using these methods, you can quickly get feedback from potential customers and determine if your product is in demand.
- Prototyping: Once an idea has been validated, it is time to create a prototype. This working product model can be used to test the functionality and usability. You can quickly create a prototype using rapid prototyping techniques and get feedback from potential customers.
- Testing: The prototype is then tested with users to get feedback. This feedback can be used to improve the product before it is launched. Using iterative testing, you can quickly make changes to the product based on user feedback.
- Development: Once the product is finalized, it is time to start development. This involves the actual manufacturing or creation of the product. By using lean manufacturing techniques, you can reduce the time it takes to develop and manufacture the product.
- Launch: Once the product is developed, it is time to launch it on the market. This involves marketing and sales activities to reach potential customers. Digital marketing techniques can quickly reach a large audience and launch your product.
It is important to note that compressing time in the new product development process can have some challenges. For example, you may need to compromise on quality or features. However, if you can successfully compress time, you can gain a significant competitive advantage. AI Marketing Plan for Manufacturing Businesses
Here are some additional tips for a compressing time in the new product development process:
- Use agile development methodologies: Agile methodologies allow you to break down the product development process into smaller, more manageable tasks, which can help you deliver the product faster. AI product management specialization and AI product management take it to another level, as we do with our industry models.
- Use cloud-based tools: Cloud-based tools can help you collaborate more effectively with team members and share files more easily. This can save you time and improve communication.
- Automate tasks: Many tasks in the new product development process can be automated, freeing up time to focus on more important tasks.
- Get feedback early and often: By getting feedback from potential customers early and often, you can quickly identify and address any problems with the product. This can help you to avoid costly delays later in the process.
By following these tips, you can reduce the time spent on the new product development process and bring your product to market faster.
Artificial intelligence (AI) can compress the time to market in a new product development process in several ways. Here are a few examples:
- Automate tasks: AI can automate many tasks involved in product development, such as data collection, analysis, and testing. This can allow product teams to focus on more creative and strategic tasks.
- Improve accuracy: AI product management can help improve market research accuracy by providing insights into user behavior and preferences. This can help to identify new opportunities and create products that are more likely to be successful.
- Reduce costs: AI can help reduce product development costs by automating tasks and making the process more efficient.
- Speed up prototyping: AI can be used to create rapid prototypes of products, which can help to shorten the product development cycle.
- Improve decision-making: AI product management can help product teams make better decisions by providing insights into data and trends. This helps ensure products are developed to meet the target market’s needs.
Overall, AI can be a valuable tool for businesses that want to compress the time to market for new products. However, it is important to note that AI is not a magic bullet. A strong product development process and using AI in conjunction with the existing process are still important.
Here are some examples of how AI compresses time to market in new product development:
- Google uses AI to help its engineers design new products. For example, Google’s AI-powered design tool, DeepDream, can help engineers visualize different design options and identify potential problems.
- Amazon uses AI to help its product managers decide which products to launch. For example, Amazon’s AI-powered product recommendation engine can help product managers identify products that are likely to be successful.
- Netflix uses AI to recommend movies and TV shows to its users. This helps Netflix keep its users engaged and increases the likelihood that they will continue to subscribe to the service.
These are just a few examples of how AI compresses time to market in new product development. As AI technology continues to develop, we can expect to see even more innovative ways to use AI to speed up the product development process.
Case Study: Harnessing AI to Drive Product Decisions at Amazon
Amazon, the world’s largest online retailer, is renowned for using advanced technologies such as AI product management and machine learning to drive business decisions.
Amazon product Managers (PMs) use AI-driven insights to decide which products to launch and how to position and market them effectively.
The Challenge:
With millions of products in its catalog and a diverse customer base, Amazon faces the daunting task of determining which products resonate with which customers. PMs must forecast demand, understand customer preferences, and assess market competition, which can be challenging in a constantly changing e-commerce environment.
The AI Solution:
Amazon uses AI to generate insights that guide PMs’ decisions in several key areas:
- Demand Forecasting: Amazon uses AI algorithms to predict product demand based on historical sales data, seasonal trends, and other factors. These forecasts help PMs decide which products to launch, when to launch them, and how many units to stock.
- Customer Segmentation and Personalization: Amazon uses AI to analyze customer behavior and segment customers into distinct groups based on their preferences and buying habits. This helps PMs understand which products will likely appeal to different customer segments.
- Market Analysis: AI algorithms analyze vast amounts of data from Amazon’s marketplace and external sources to assess market trends and competition. This helps PMs understand the market landscape and make informed product positioning and pricing.
- Product Recommendations: Amazon’s recommendation engine uses AI to suggest products to customers based on their past purchases, browsing history, and other factors. This boosts existing product sales and helps PMs gauge customer interest in new products.
Results:
The use of AI has significantly improved the decision-making process for PMs at Amazon:
- Improved Accuracy: AI-driven demand forecasts have proven more accurate than traditional methods, reducing overstock and stockout situations.
- Customer Satisfaction: Personalized product recommendations have enhanced the customer shopping experience, leading to higher customer satisfaction and repeat purchases.
- Increased Efficiency: By automating complex analyses, AI has freed PMs to focus more on strategic decisions and less on data crunching.
- Enhanced Product Performance: AI has helped Amazon launch products that are more likely to succeed in the market.
Amazon’s case demonstrates how AI can revolutionize product management. AI empowers PMs to make data-driven decisions more efficiently and accurately by providing valuable insights and automating complex analyses.
As AI technology advances, it’s likely to play an increasingly vital role in product management.
An AI-driven product development process is superior to traditional product development processes because it harnesses data, automates repetitive tasks, enhances decision-making, and improves product outcomes.
Explanation:
- Data-Driven Insights: AI product management can process vast amounts of data at high speeds, uncovering patterns, trends, and insights that would be difficult for humans to detect. This can inform everything from market analysis to product design, making the development process more evidence-based and less reliant on guesswork or intuition.
- Automated Tasks: AI can automate many repetitive and time-consuming tasks in product development, such as data collection and analysis, customer research, or even certain aspects of product testing. This can make the development process more efficient and allow product teams to focus more on strategic and creative tasks.
- Enhanced Decision Making: AI can enhance decision-making in product development by providing predictive analytics, decision support tools, and real-time feedback. For example, AI could predict how changes in a product feature might impact customer satisfaction or sales, helping product teams make more informed decisions.
- Improved Product Outcomes: By providing data-driven insights, automating tasks, and enhancing decision-making, AI product management has the potential to improve the outcomes of the product development process, leading to more successful products in the market.
Advantages in Table Format:
Advantages for AI-Driven Product Development
Traditional Product Development | AI-Driven Product Development | |
Data-Driven Insights | Relies heavily on human analysis and interpretation | Uses algorithms to process large amounts of data quickly |
Automated Tasks | Manual execution of repetitive tasks | Automates repetitive, time-consuming tasks |
Enhanced Decision Making | Decisions based on experience, intuition, and available data | Decisions augmented with AI predictive analytics and real-time feedback |
Improved Product Outcomes | Success dependent on multiple variables, with a risk of human error | Improved outcomes due to data-driven insights, automation, and enhanced decision-making |
Conclusion about AI product management:
While traditional product development methods have proven effective, integrating AI product management into the process brings a new level of efficiency, accuracy, and data-driven decision-making.
By harnessing the power of AI, organizations can optimize their product development process, making it more efficient and likely to result in successful products.
However, as with any technology, it is important to remember that AI is a tool that aids the process and not a replacement for human creativity, strategic thinking, and leadership.
General FAQs on AI product management
Can AI Product Management help make better product decisions?
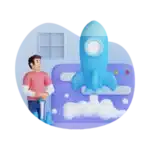
Yes, AI Product Management enhances decision-making capabilities by providing data-driven insights, predictive analytics, and real-time feedback. AI tools can predict how changes in product features might impact customer satisfaction, sales, or market performance. These insights can guide product managers to make informed decisions that align with the company’s goals and customer needs.
How does AI Product Management improve efficiency in the product development process?
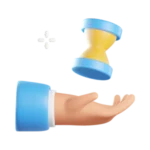
AI Product Management can automate repetitive and time-consuming tasks, such as data collection, customer research, or even certain aspects of product testing. This automation allows product teams to focus more on strategic decisions and creative tasks, increasing overall efficiency. Additionally, AI-driven predictive analytics can facilitate quicker decision-making, reducing time spent on deliberation.
How does AI Product Management improve product outcomes?
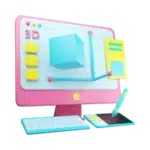
By offering data-driven insights, automating mundane tasks, and improving decision-making, AI can enhance the outcomes of the product development process. AI’s precise data analysis and predictive capabilities help product managers better understand the market and customer needs, design more appealing features, and anticipate potential challenges. This makes products more likely to succeed in the market, improving overall performance.
How does AI Product Management provide more precise data analysis than traditional methods?
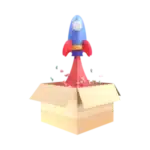
AI Product Management leverages machine learning and advanced algorithms to process and analyze vast amounts of data more efficiently and accurately than human analysis. This ability can unearth deep insights, trends, and patterns that are not immediately apparent with traditional data analysis methods. Such precise data analysis allows for better decision-making throughout product development, from identifying customer needs to predicting product performance.